23.01.2023 Blogbeitrag beim Kompetenznetzwerk für automatisierte und vernetzte Mobilität
Ein Blogbeitrag zum Thema „Wie Künstliche Intelligenz die Vorhersage kritischer Situationen beim automatisierten Fahren präzisiert" wurde beim Kompetenznetzwerk für automatisierte und vernetzte Mobilität in Nordrhein-Westfalen veröffentlicht.
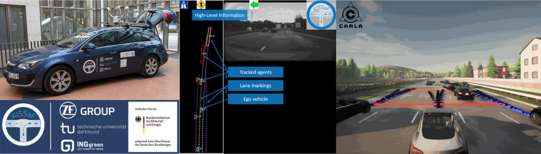
20.12.2022 Youth-Author Award für Wissenschaftler Projekts KISSaF
Unsere Forscher wurden vom VDI-Fachausschuss Computational Intelligence für ihren Beitrag "Conditional Behavior Prediction for Automated Driving on Highways" mit dem Jugend-Autoren-Preis 2022 ausgezeichnet.
Die Vorhersage des Verhaltens von Agenten in Multi-Agenten-Systemen ist entscheidend für sicheres automatisiertes Fahren. Unsere Arbeit zeigt, wie die Einbeziehung von High-Level-Routeninformationen die Vorhersageleistung in Autobahnszenarien verbessert. Darüber hinaus kann der Ansatz auch zur Vorhersage der zukünftigen Bewegung des selbstfahrenden Fahrzeugs verwendet werden und als lernbasiertes Bewegungsplanungsmodul dienen.
Diese Forschung wurde gefördert durch das Bundesministerium für Wirtschaft und Klimaschutz aufgrund eines Beschlusses des Deutschen Bundestages.
29.09.2022 Poster zum „Mid-term status report on KISSaF“ wird auf der AmE 2022 präsentiert
Vom 29. bis zum 30. September 2022 findet die AmE (Automotive meets Electronics) 2022
in den Westfalenhallen in Dortmund statt.
Auf der Konferenz wird in der Session 4 des Programms das Poster „Mid-term status report on KISSaF: AI-based situation interpretation for automated driving“ präsentiert.
Weitere Informationen finden Sie auf dem Poster und in dem ausführlicheren Paper.
08.09.2022 Postervorstellung auf dem 17.DAT
Das KISSaF-Projekt wurde mit einem Poster und einem Kurzvortrag auf dem 17. DortmunderAutoTag vorgstellt.
09.06.2022 Midterm presentation of the project
On June 09, 2022, in the Rudolf Chaudoire Pavilion of the TU Dortmund University, the midterm presentation of the project KISSaF - AI-based Situation Interpretation for Automated Driving. In addition to scenario prediction, other topics such as vehicle design, perception, learning-based planning and evaluation were addressed in several presentations. We want to thank all participants for their contributions through presentations and discussions. At the hybrid event, we welcomed 28 people each in presence and online.
19.01.2022 Best Paper Award at NeuRIPS Workshop
Our contribution "UMBRELLA: Uncertainty-Aware Model-Based Offline Reinforcement Learning Leveraging Planning" achieved the best paper award at the NeuRIPS 2021 Workshop Machine Learning for Autonomous Driving.
While showing promising results in simulated environments, online reinforcement learning is often still not applicable to safety-critical applications due to the nature of trial and error learning. In the offline reinforcement learning setting, the agent can learn from a previously collected dataset and does not have to interact with the environment.
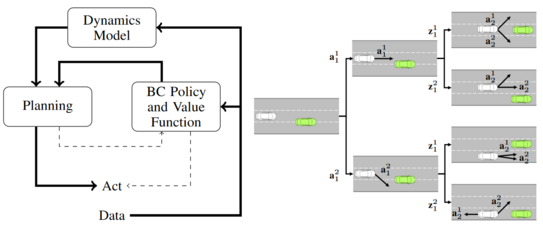
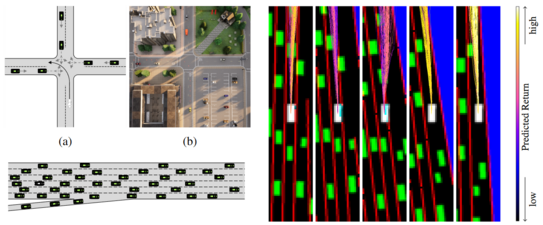
The developed approach solves the prediction planning and control problem of automated in multi-agent environments. It accounts for aleatoric and epistemic uncertainty. The interaction between prediction and planning is modeled by a learned action-conditioned stochastic dynamics model, which predicts the next state based on the previous state, the current action, and a sampled latent variable. A behavior-cloned policy guides the action sampling. By sampling different latent variables, we can predict different future scene evolutions. We roll out multiple future scene evolutions and then choose the optimal action using a stochastic optimization technique. This model-based approach results in high interpretability
Workshop: https://ml4ad.github.io/
Paper: https://arxiv.org/abs/2111.11097
This research was supported by the Federal Ministry for Economic Affairs and Energy on the basis of a decision by the German Bundestag.
30.04.2021
Erste Pressemitteilung auf der Webseite der TU-Dortmund.