01/23/2023 Blog post at the Competence Network for Automated and Connected Mobility A blog post on "How Artificial Intelligence Precises the Prediction of Critical Situations in Automated Driving" was published at the Competence Network for Automated and Connected Mobility in North Rhine-Westphalia.
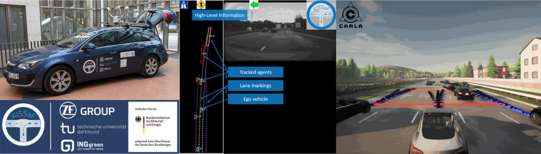
20.12.2022 Youth-Author Award for Scientists Project KISSaF
Our researchers have been awarded the 2022 Youth Author Award by the VDI Computational Intelligence Expert Committee for their paper"Conditional Behavior Prediction for Automated Driving on Highways". Predicting the behavior of agents in multi-agent systems is critical for safe automated driving. Our work shows how incorporating high-level route information improves prediction performance in highway scenarios. Furthermore, the approach can also be used to predict the future movement of the self-driving vehicle and serve as a learning-based movement planning module.
This research was funded by the German Federal Ministry of Economics and Climate Protection based on a resolution of the German Bundestag.
29.09.2022 Poster on "Mid-term status report on KISSaF" to be presented at AmE 2022
AmE (Automotive meets Electronics) 2022 will be held at the Westfalenhallen in Dortmund, Germany, from September 29 to 30, 2022. At the conference, the poster "Mid-term status report on KISSaF: AI-based situation interpretation for automated driving" will be presented in session 4 of the program.
More information can be found on the poster and in the more detailed paper.
08.09.2022 Poster presentation at the 17.DAT
The KISSaF project was presented with a poster and a short talk at the 17th DortmunderAutoTag.
09.06.2022 Midterm presentation of the project On June 09, 2022, in the Rudolf Chaudoire Pavilion of the TU Dortmund University, the midterm presentation of the project KISSaF - AI-based Situation Interpretation for Automated Driving. In addition to scenario prediction, other topics such as vehicle design, perception, learning-based planning and evaluation were addressed in several presentations. We want to thank all participants for their contributions through presentations and discussions. At the hybrid event, we welcomed 28 people each in presence and online.
19.01.2022 Best Paper Award at NeuRIPS Workshop
Our contribution "UMBRELLA: Uncertainty-Aware Model-Based Offline Reinforcement Learning Leveraging Planning" achieved the best paper award at the NeuRIPS 2021 Workshop Machine Learning for Autonomous Driving.
While showing promising results in simulated environments, online reinforcement learning is often still not applicable to safety-critical applications due to the nature of trial and error learning. In the offline reinforcement learning setting, the agent can learn from a previously collected dataset and does not have to interact with the environment.
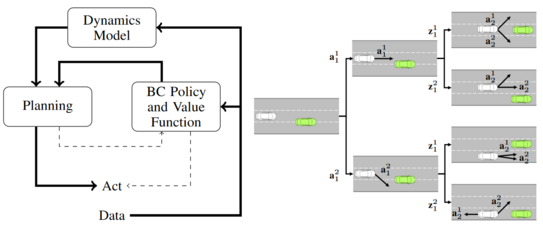
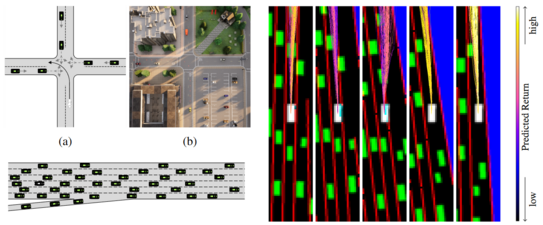
The developed approach solves the prediction planning and control problem of automated in multi-agent environments. It accounts for aleatoric and epistemic uncertainty. The interaction between prediction and planning is modeled by a learned action-conditioned stochastic dynamics model, which predicts the next state based on the previous state, the current action, and a sampled latent variable. A behavior-cloned policy guides the action sampling. By sampling different latent variables, we can predict different future scene evolutions. We roll out multiple future scene evolutions and then choose the optimal action using a stochastic optimization technique. This model-based approach results in high interpretability
Workshop: https://ml4ad.github.io/ Paper: https://arxiv.org/abs/2111.11097
This research was supported by the Federal Ministry for Economic Affairs and Energy on the basis of a decision by the German Bundestag.
30.04.2021
First press release on the TU-Dortmund website.